- The AI Citizen
- Posts
- The Dangers of AI Training on AI-Generated Content
The Dangers of AI Training on AI-Generated Content
Understanding Model Collapse
As AI becomes increasingly woven into the fabric of our daily lives, a critical question arises: What happens when AI models are trained on data that they, or other AI systems, have generated? This scenario is akin to making a copy of a copy—the quality deteriorates over time, leading the AI further away from accurate representations of reality.
The Phenomenon of Model Collapse
This degradation is not just theoretical; it’s a documented phenomenon known as “model collapse”. In their 2023 paper titled “The Curse of Recursion: Training on Generated Data Makes Models Forget,” the researchers explored how AI models trained on outputs from other AI systems suffer from reduced diversity and increased distortion in their outputs. They observed that:
“The model becomes poisoned with its own projection of reality.”
Key Findings from Top Studies
1. Loss of Diversity and Originality: Models begin to produce repetitive and less diverse outputs when trained on AI-generated data. This was highlighted in a study published in the Proceedings of the National Academy of Sciences, where researchers found that recursive training leads to homogenization of language patterns.
2. Amplification of Errors: Small inaccuracies in the initial AI-generated data can become significantly magnified. A paper from MIT Technology Review discussed how errors compound over iterations, leading to outputs that deviate substantially from factual information.
3. Bias Reinforcement: AI models can inadvertently reinforce and amplify biases present in AI-generated content. The Journal of Artificial Intelligence Research published findings showing that models trained on biased data produce even more skewed results.
4. Erosion of Knowledge: According to a study by OpenAI, models can “forget” factual information over time when trained recursively on their own outputs, a phenomenon known as catastrophic forgetting.
5. Difficulty in Model Correction: As errors and biases accumulate, it becomes increasingly challenging to retrain models to correct these issues without extensive intervention.
The Risk of a Contaminated Data Pool
The internet serves as a vast repository of information, and AI models often rely on this data for training. However, with the surge of AI-generated content online—from automated news articles to machine-translated texts—the risk of AI models inadvertently training on AI-generated data increases.
Real-World Examples
• Automated News Generation: Several media outlets use AI to generate news articles. If these AI-written articles become part of the training data for new models, inaccuracies or stylistic quirks can be propagated and amplified.
• Machine Translation Loops: Platforms that rely on AI for translation may introduce errors. If these translated texts are used as training data, the AI learns from its own mistakes.
• Content Farms: Websites generating large volumes of AI-written content to boost SEO rankings flood the internet with low-quality information. This content can contaminate training datasets.
The Destructive Feedback Loop
1. AI Generates Content: AI systems produce a significant portion of online content.
2. Content Enters Data Pool: This content becomes part of the data pool that future AI models use for training.
3. Training on AI Data: New AI models train on this data, which may lack the nuance and accuracy of human-generated content.
4. Degraded Outputs: The new models produce even less accurate or diverse outputs.
5. Cycle Repeats: The process repeats, amplifying inaccuracies and biases over time.
Implications for AI Development and Society
The consequences of model collapse extend beyond technical performance. If AI systems propagate inaccuracies or biases present in AI-generated training data, this can affect applications ranging from search engines to decision-making algorithms in finance or healthcare.
Practical Impacts
• Healthcare Diagnostics: AI models used for diagnosing diseases could make erroneous recommendations if trained on flawed data, leading to misdiagnoses.
• Financial Algorithms: Trading bots and financial forecasting models might make poor investment decisions, affecting markets and economies.
• Legal and Judicial Systems: AI used in legal contexts could perpetuate biases, leading to unfair sentencing or legal advice.
Case Study: The Chatbot Echo Chamber
Consider a chatbot designed to provide historical information. If it begins to train on transcripts of conversations that include its own prior outputs, any inaccuracies it previously provided can become “facts” in its training data. Over time, the chatbot’s knowledge base becomes polluted, and it starts offering increasingly distorted historical accounts.
Mitigating the Risks
To address these challenges, researchers and developers can implement several strategies.
Curate Training Data
• Human Oversight: Involve human reviewers to ensure the quality of training data.
• Source Verification: Use data from reputable and verified sources to minimize inaccuracies.
Detect and Filter AI-Generated Content
• Content Tagging: Implement markers in AI-generated content to identify it easily.
• Algorithmic Filters: Develop algorithms that can detect and exclude AI-generated data from training sets.
Continuous Monitoring and Updating
• Performance Audits: Regularly test AI models for signs of degradation or bias.
• Feedback Loops: Incorporate user feedback to correct and improve model outputs.
Establish Ethical Guidelines
• Industry Standards: Create guidelines for AI training practices to prevent recursive training pitfalls.
• Transparency: Encourage openness about the sources of training data and the methods used.
Conclusion
As consumers of digital content, it’s important to remain aware that not all information is created equal. The content we read online might not just be AI-generated—it could be the product of AI models trained on AI-generated data, potentially amplifying inaccuracies. Recognizing and addressing the risks of model collapse is crucial for the responsible development of AI technologies.
By implementing robust training practices and continuously monitoring AI outputs, we can mitigate the risks associated with training AI on AI-generated content. This ensures that AI remains a tool that enhances human capabilities rather than diminishing them.
This article was written by a human to raise awareness about the challenges of AI training on AI-generated content.
Sponsored by World AI X
The CAIO Program: Preparing Executives to Lead Their Organizations and Sectors in the AI Era
Next Kickoffs: October 21 ; November 18
World AI X is excited to extend a special invitation for executives and visionary leaders to join our Chief AI Officer (CAIO) program! This is a unique opportunity to become a future AI leader or a CAIO in your field.
During a transformative, live 6-week journey, you'll participate in a hands-on simulation to develop a detailed AI strategy or project plan tailored to a specific use case of your choice. You'll receive personalized training and coaching from the top 1% industry experts who have successfully led AI transformations in your field. They will guide you through the process and share valuable insights to help you achieve success.
7 Unique Features That Make The CAIO Program Stand Out:
Personalized Experience: The program is tailored to your profile and needs, ensuring you become an AI leader in your domain.
Proprietary AI Leadership Frameworks: Learn our unique, practical frameworks and a step-by-step approach to developing a detailed AI strategy for your organization.
Expert Coaching: Get matched with top coaches and industry experts in your sector who have successfully navigated their AI transformation journeys.
Organizational Impact: Develop an AI strategy that enhances your organization’s competitiveness and market valuation.
Leadership Advancement: Boost your visibility by publishing high-quality articles, presenting at the World AI Forum, and gaining exposure as a member of the World AI Council.
Networking Opportunities: Connect and collaborate with other top executives and peers, building a supportive community for your AI journey.
Exclusive World AI Council Membership: Receive a complimentary 1-year membership to the World AI Council, providing access to exclusive resources, quarterly reports, and opportunities to speak at our annual World AI Forum.
Exclusive Offer
By enrolling in the program, candidates can attend any of the upcoming cohorts over the next 12 months, allowing multiple opportunities for learning and growth.
We’d love to help you take this next step in your career.
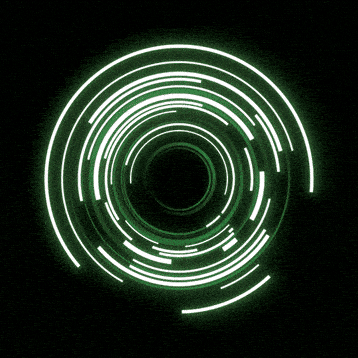
Gif by xponentialdesign on Giph
Sponsored by World AI X
AI Basics in 60 Minutes
Are you curious about AI? Jump into our free 60-minute AI Basics course! It's perfect for beginners and a great way to start chatting about AI like a pro. Join us, learn quickly, and enjoy the conversation—sign up now and explore AI with friends!
About The AI Citizen Hub - by World AI X
This isn’t just another AI newsletter; it’s an evolving journey into the future. When you subscribe, you're not simply receiving the best weekly dose of AI and tech news, trends, and breakthroughs—you're stepping into a living, breathing entity that grows with every edition. Each week, The AI Citizen evolves, pushing the boundaries of what a newsletter can be, with the ultimate goal of becoming an AI Citizen itself in our visionary World AI Nation.
By subscribing, you’re not just staying informed—you’re joining a movement. Leaders from all sectors are coming together to secure their place in the future. This is your chance to be part of that future, where the next era of leadership and innovation is being shaped.
Join us, and don’t just watch the future unfold—help create it.
For advertising inquiries, feedback, or suggestions, please reach out to us at [email protected].
Reply